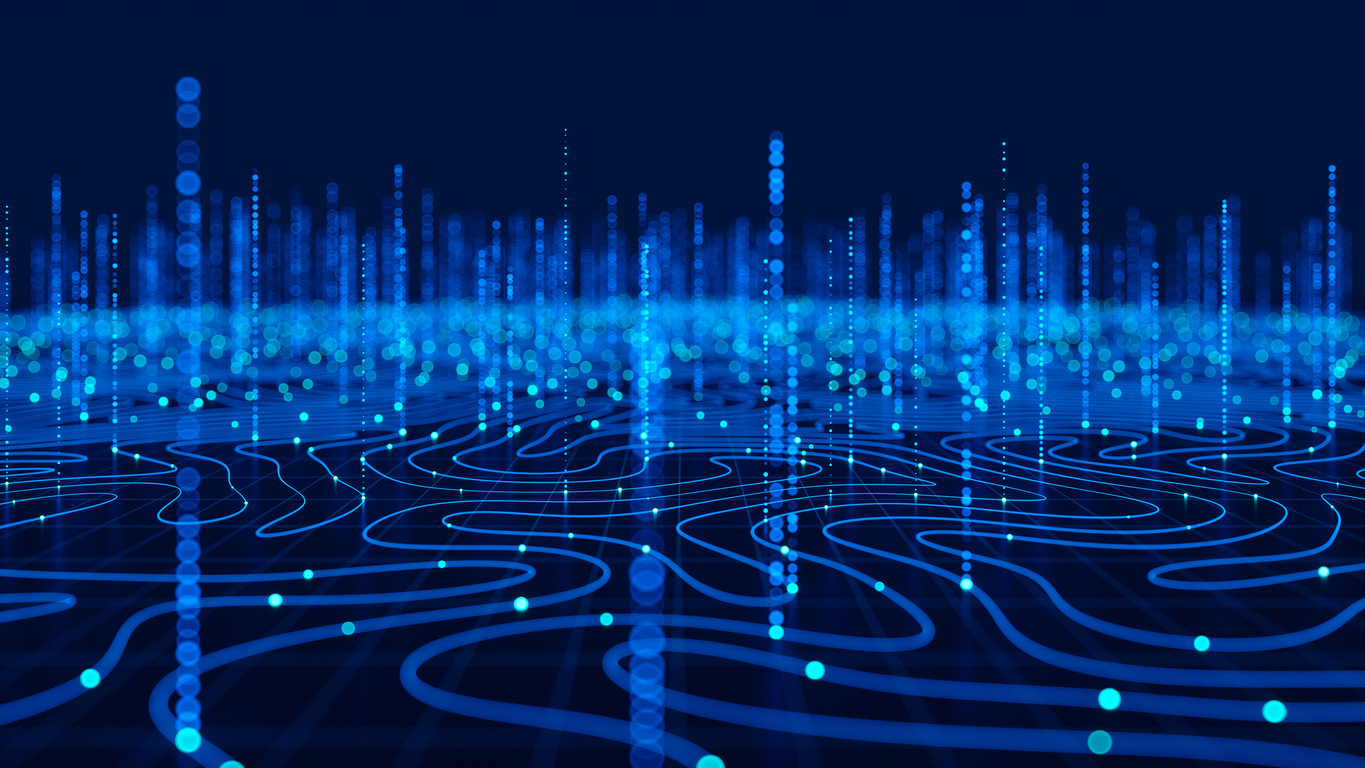
The Department of Health and Human Services piqued interest last month with notification that its case use inventory for artificial intelligence (AI) had tripled in the last year. HHS’s listed cases uses point to how its agencies hope to leverage AI’s potential while navigating federal policies, stakeholder skepticism, and technological challenges.
HHS’s 2023 inventory, publication of which was required under an executive order passed during the Trump Administration, lists 163 non-classified and non-sensitive current and planned AI use cases across seven agencies.
The National Institutes of Health (NIH) led the list with 47 use cases. The Food and Drug Administration (FDA) was a close second with 44 use cases.
Many of the applications merely streamline clerical and administrative workflows. For example,a chatbot may soon replace a public inquiry phone line at the Administration for Health Research and Quality (AHRQ). However, others suggest an increasing integration of AI in the sub-agencies’ more sophisticated data gathering and decision-making processes.
Defining AI
The term AI is used to reference technologies which allow machines to perform cognitive tasks once considered to be the exclusive domain of humans. HHS and other federal offices employ the definition offered by the National Security Commission on Artificial Intelligence, which begins with the characterization of AI as “any artificial system that performs tasks under varying and unpredictable circumstances without significant human oversight, or that can learn from experience and improve performance when exposed to data sets.”
For HHS, AI must be “TAI,” an expanded acronym specifying that the AI must be “trustworthy.”
In keeping with Executive Order (EO) 13960 Promoting the Use of Trustworthy Artificial Intelligence in the Federal Government, the Department’s TAI playbook specifies that any AI employed by HHS sub-agencies needs to include safeguards to protect privacy, civil rights, civil liberties, “and American values, consistent with applicable laws.” In pursuit of this goal, the department established an Office of the Chief Artificial Intelligence Officer (OCAIO) and appointed its first chief officer in 2021.
AI encompasses many processes. HHS’s inventory shows that it is exploring a variety of them.
Machine learning and natural language processing
In the age of ChatGPT and Bard, much of what is referred to as AI is actually its subfield of machine learning (ML). In ML, computers or machines learn on their own in much the same way as human. One of the most familiar examples of ML is natural language processing (NLP) in which machines learn to understand language(s).
NLP, the technology behind Siri and Alexa, is also fueling the chatbots which HHS notes will be employed by AHRQ, NIH, and the FDA’s Office of Science Customer Assistance Response.
Of greater interest may be the FDA’s use of NLP in its Term Identification and Novel Synthetic Opioid Detection and Evaluation Analytics launch. Under the program, the FDA will analyze social media for emerging drug terminology and references in much the way businesses use AI to monitor social media posts for consumer preferences and behavior.
The FDA’s Center for Drug Evaluation and Research’s Office of New Drugs is employing NPL in conjunction with data mining to detect and better understand drug-induced adverse events (AEs) in marketed drugs. Similarly, the FDA’s Center for Biologics Evaluation and Research, in collaboration with CBER/OBPV/DABRA is labeling and pulling biologics-related adverse events from electronic health records (EHRs).
NIH is using NLP in at least eight programs related to its grants processes. These include an HIV-related grant classifier tool with interactive data visualization.
And even as NIH employs AI to manage grant processes, HHS’s Office of the Inspector General has been using it in audit processes to identify potential anomalies between grantees.
Managing large data sets
Medicare and its contractors process over one billion claims annually; even small percentages of fraud in the system can quickly cost U.S. taxpayers millions of dollars. Unfortunately, those who have previously defrauded government payment programs say that doing so has become comparatively easy.
However, the sheer volume of claims data makes Medicare an ideal opportunity for the Centers for Medicare & Medicaid Services (CMS) to deploy another ML process known as the random forest technique.
As the name implies, random forests entail the combination of multiple decision trees and the aggregation of their predictions. They are especially well suited for large data sets.
Meanwhile other unspecified ML processes are being used in the Medicaid and Children’s Health Insurance Program (CHIP) to identify outliers in data submitted by disproportionate share hospitals (DSHs), while the Medicaid and CHIP Financial (MACFin) is exploring an AI forecasting model to predict future DSH payments.
Neural networks and deep learning for image recognition
The MI subsets of neural networks and deep learning, in which a network of millions of processing nodes can be interconnected, has given machines the ability to recognize images. The Centers for Disease Control and Prevention’s (CDC’s) National Center for Chronic Disease Prevention and Health Promotion (NCCDPHP) Division of Diabetes Translation (DDT) is investigating whether this technology could eventually replace ophthalmologists in grading retinal photos collected as part of the National Health and Nutrition Examination Survey (NHANES).
Those for whom discussions of AI conjure Orwellian visions may find a degree of validation in the CDC’s plan to develop and promote techniques for inputting image-based data specific to community infrastructure. But, if Big Brother is watching, in this case it is only to identify sidewalks and bicycle lanes as opportunities for exercise.
Challenges
There has been much recent discussion of the potential of AI to transform healthcare. HHS’s case use inventory provides a glimpse into how it could transform the agencies regulating healthcare in the U.S.
Yet, success is not guaranteed.
Implementing AI processes is not simply a matter of “plug and play.” In addition to substantial computational capacity, deep learning takes tremendous amounts of data.
Because successful AI also requires “smart data,” agencies will need to budget time and resources to build high-quality data sets before overlaying AI processes. In fact, the description of MACFin’s DSH payment forecasting model includes a notation that over six years’ worth of data needed to be “cleaned” and “combed” for the project.
Without good data, AI can perpetuate existing biases and inequities in healthcare delivery. Similarly, AI algorithms using inappropriate proxies can reach wildly inaccurate conclusions. As an example, a model using healthcare expenditures as a proxy for health status concluded that Black Americans were healthier because less money was spent on their healthcare. It did not allow for the possibility that systemic biases prevented Black Americans from receiving the care they needed.
While AI is good at sorting through images, it is best at describing things it has previously encountered. Outliers can stump it. In an amusing example, photos of a rare spotless giraffe born at a Tennessee zoo engaged humans, but challenged bots asked to describe it.
Workforce implications
Adoption of AI has a very real potential to transform the human resources profile of HHS agencies, which currently have an attrition rate below the federal average.
One need only look to statements from the striking Writers Guild of American (WAG) and Screen Actors Guild-American Federation of Television and Radio Artists SAG-AFTRA for evidence that many Americans consider AI an existential threat.
Remarkably, some AI developers concur. In testimony before the Senate Judiciary Committee in May, Sam Altman, co-founder and CEO of OpenAI, the company behind ChatGPT, said “that new AI tools can have profound impacts on the labor market.”
Research suggests that these impacts will take a form not previously seen before.
While previous technological advances have traditionally displaced less educated workers through the automation of processes, AI poses a threat to knowledge workers. According to a McKinsey report, “Generative AI is likely to have the biggest impact on knowledge work, particularly activities involving decision making and collaboration, which previously had the lowest potential for automation.”
Conclusion
It is reasonable to expect that HHS’s annual AI case use inventory will continue to grow and that, with each year, it will include a wider variety of AI processes. HHS will need to ensure that technologies further the department’s mission without compromising its values and, like other public and private entities, it will need to prepare for the repercussions from a disrupted HR landscape.
The use of AI may ultimately become so commonplace that inventories are no longer required. For now, they provide an important glimpse into a sector in transition.