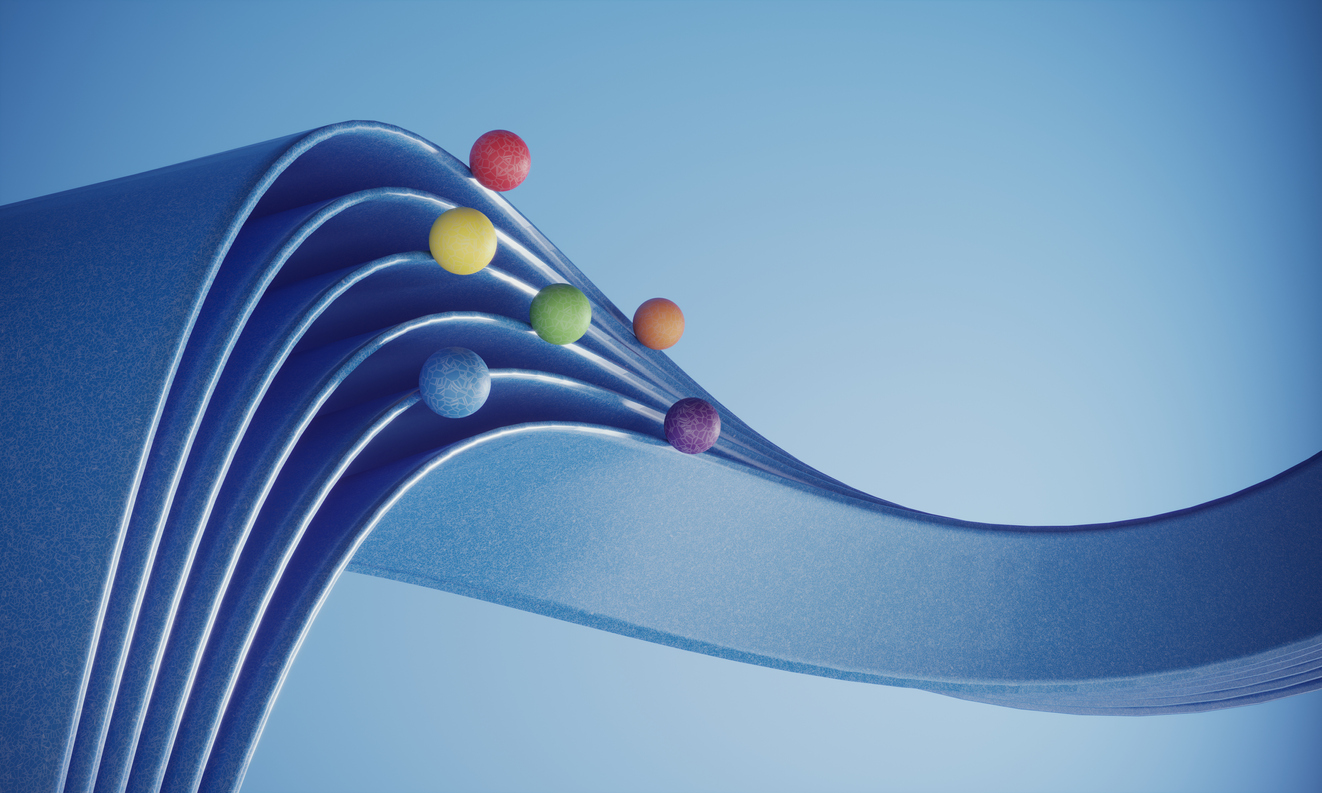
As the United States transitions from a fee-for-service (FFS) model of healthcare delivery to value-based payments (VBP), both payers and providers have recognized the importance of fairly accounting for preexisting differences in patient health status.
The management of heart disease in a patient with diabetes and high blood pressure is inherently more complex and consequently more costly than treating the same condition in a patient without comorbidities. In the absence of a method to adjust for these variances, VBP becomes inequitable, penalizing providers who care for sicker patient populations.
To address these differences in patient health status, payers employ risk adjustment—a methodological approach intended to level the playing field in VBP assessments of outcomes, expenditures, and performance.
However, risk adjustment models introduce distinct challenges. As incentives within VBP have been reconfigured, some health plans and providers have learned to manipulate risk adjustments for financial gain. Moreover, emerging research suggests that poorly designed risk adjustments can perpetuate inequities in healthcare.
Background
The FFS model, which dominated U.S. healthcare through the beginning of this century, incentivized the utilization—and many argued, overutilization—of medical services and procedures. Critics contended that volume took precedence over quality. With provider reimbursement directly linked to consumption, tests were ordered, procedures scheduled, beds filled, and services billed without requirements to document value or efficiency.
VBP models were introduced as a method for shifting payment from being tied to service consumption to being linked to patient health outcomes. Framed as a means for improving care while curbing expenditures, VBP represented a dramatic realignment of financial incentives.
Today, VBP models, including Managed Care Organizations (MCOs), Accountable Care Organizations (ACOs), and the Medicare Advantage (MA) program, are commonplace in healthcare. Each incorporates risk adjustments to account for differences in patients’ health status.
Determining risk adjustment scores
Risk adjustment requires both extensive data collection and advanced analytics to link metrics with outcomes.
Arguably, the most notable example of a risk adjustment matrix is the Centers for Medicare & Medicaid Services’ (CMS’s) Hierarchical Condition Categories (CMS-HCC). With inputs for both demographic and clinical data, CMS-HCC enables the calculation of a risk adjustment factor (RAF) for each MA beneficiary.
CMS-HCC is prospective and uses diagnoses from one year to predict healthcare expenses in the next.
Coding creep
Realigning incentives in healthcare is not the same as eliminating them. Not surprisingly, as the basis for payment has been redefined, health plans and providers have adjusted their strategies in pursuit of new financial targets.
When compensation is tied to the complexity and severity of patient conditions, providers may deliberately assign higher-severity diagnosis codes to patients in order to inflate the perceived level of patient risk. Empirical and anecdotal evidence suggests that such “upcoding” or “coding intensity” has become commonplace.
In one study, MA plan enrollees were found to have six to 16 percent higher diagnosis-based risk scores than what would have been anticipated under FFS Medicare. With higher risk scores leading to higher payments, ICD-10 codes which might not be included in patients’ records in traditional Medicare models take on a new importance under MA’s payment matrix.
The potential financial advantage derived from favorable risk adjustments has led to the emergence of a niche market for “risk adjustment optimization” software. In addition, managed care plans contracted with government payors often employ tactics designed to encourage providers to maximize diagnoses. These might include requiring evaluation visits, prepopulating notes with codes from previous visits, and specifically requesting changes in coding.
While seeking to optimize risk adjustment is lawful, government agencies, private payers, and regulatory bodies are vigilant in monitoring for instances in which efforts to maximize payments veer into fraudulent practices. Organizations found guilty of upcoding can face serious consequences, including financial penalties and reputational injury.
The Department of Justice and the Department of Health and Human Services (Hhave jointly acknowledgedthat billions of dollars in estimated MA payments have been made based solely on annual health risk assessments (HRAs), with no diagnosis-specific care provided and no supporting claims data.
Providers may also conduct “one-way audits” to serve their financial interests by incorporating valid diagnoses that raise patients’ risk scores without correcting erroneous diagnoses that might lower them. While such practices might increase risk adjustment scores, they also make the offending party liable under the False Claims Act.
Assuring accuracy in risk adjustments has become an ongoing struggle for regulators. CMS strives to identify improper payments Medicare Advantage Organizations through Risk Adjustment Data Validation (RADV) audits. But the Center for Medicare and Medicaid Innovation (CMMI) describes RADV audits as “expensive and time-consuming for CMS” and burdensome for plans and providers.
Accounting for SDoHs in risk adjustment
The discussion of how to fine-tune risk adjustments is longstanding. But it has taken on a new dimension with the growing appreciation for the importance of the social determinants of health (SDOHs).
SDOHs, including education, employment opportunities, housing conditions, access to healthcare, and community resources, can significantly influence an individual’s health outcomes. Yet, until recently, they were seldom integrated into VBP risk adjustments. Critics, including the Commonwealth Fund, argue that by overlooking social risk factors, risk adjustments perpetuate health disparities.
In fact, the failure to consider SDOHs may lead providers to opt out of VBP programs in specific areas.
A study published this year in Health Affairs found that zip codes with populations characterized by higher percentages of Black residents, impoverished individuals, those without insurance, or those with less than a high-school education were less likely to be served by an ACO than zip codes with predominantly White and well-educated residents.
Current VBP models were conceived without an appreciation for SDOHs.
In a 2020 report to Congress, HHS’s Office of the Assistant Secretary for Planning and Policy acknowledged that there is no consistent approach within the Medicare program for identifying or measuring social risks. While CMS-HCC takes into account a beneficiary’s Medicaid and disability status, it does not incorporate factors such as transportation access, nutrition, housing, and income, despite the well-documented impact of these factors on health status.
The tide may be turning. CMMI is currently exploring VBP models which incorporate both clinical diagnosis and social risks, and several states have begun to examine how to integrate SDOHs into VBP-based Medicaid plans.
Last December, Blue Cross Blue Shield of Massachusetts announced the introduction of VBP contracts including SDOH and equity measures under a program which will be independently assessed by The Center for Healthcare Organization and Innovation Research (CHOIR) at the UC Berkeley School of Public Health.
Accounting for SDOHs will be challenging. Before any single factor can be given a weight in a program’s risk adjustment formula, there will need to be agreement as to which of multiple social risks should be quantified.
The Institute of Medicine, now known as the National Academy of Medicine, previously proposed that standardized social data be integrated into electronic health records. While individual screenings for social risks provide valuable opportunities for healthcare providers to connect patients with community resources, identifying SDOHs at the individual patient level may not be practical for risk adjustment scoring.
An alternative approach involves assessing social risks at regional levels, akin to how some countries employ “deprivation indices” to assess community needs. One potential starting point for this approach is the Social Vulnerability Index (SVI) developed by the Centers for Disease Control and Prevention. Originally designed to predict community vulnerability in the face of disasters, the SVI utilizes 16 census variables to characterize each county in the United States across dimensions such as Socioeconomic Status, Household Characteristics, Racial & Ethnic Minority Status, and Housing Type & Transportation.
Much like a limited understanding of the significance of SDOHs prevented their inclusion in original risk adjustment formulas, an appreciation for their complexity and complex interplay may complicate their incorporation now.
Conclusion
Risk adjustment remains a cornerstone of VBP in healthcare. Properly modeled, it can ensure that reimbursement is equitable and accurately reflects the provision of care to diverse patient populations. But fine tuning it to accurately reflect multiple healthcare variables while limiting opportunities to “game the system” remains an ongoing challenge.